How Is Data Science Driving Modern Business Strategy? The 2023 Guide
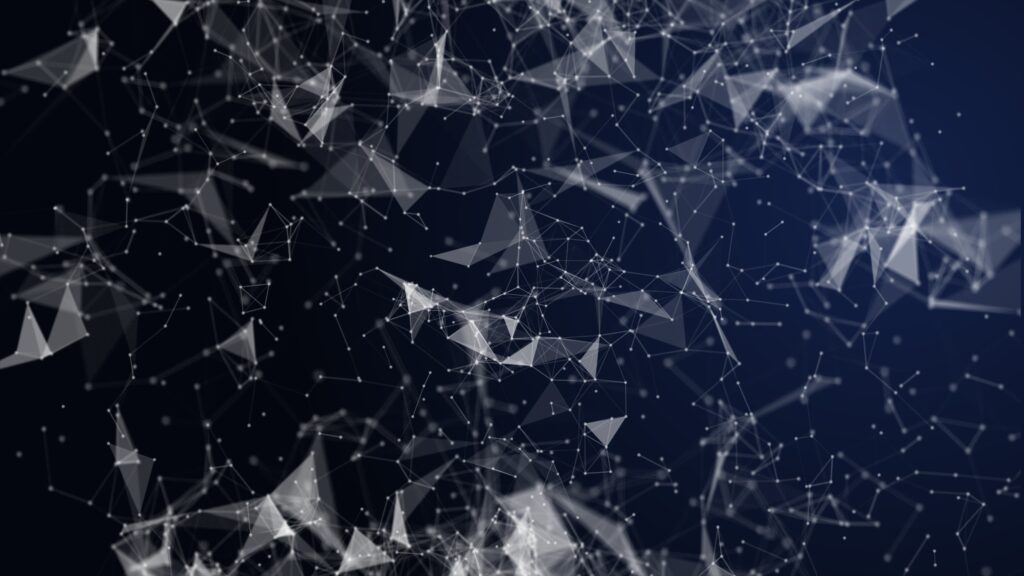
Introduction
The term ‘Data Science’ was coined in 1960 for an upcoming field of professionals whose job was to comprehend the growing volume of data amassed at the time and then turn it into insights. By 1970, with the advent of databases, real data evolution had already begun, and with it, a data revolution.
Businesses in 2023 are no longer asking if data science plays an important role but how to leverage it to formulate data-driven decisions. Data science and analytics have become a cornerstone of modern business strategy. Today, data scientists often collaborate across organizational units on strategies to maximize business performance, acquire more clients and improve retention.
Leaders across every sector are grappling with the implications (and the opportunities) of big data. In a report by Raconteur, worldwide, people will generate 463 exabytes of data per day by 2025! According to another report, the data science market was valued at $95.31 bn in 2021 and is expected to clock an impressive growth at an eye-popping CAGR of 27.6%! Netting out those statistics , the former presents the challenge, while the latter indicates that leaders are adopting data science to navigate them. This blog lists a few ways data science supports modern business strategy.
4 Ways in Which Data Science Is Revolutionizing Modern Business Strategy
1.Personalizing Products & Services For Individual Customers
Big data, coupled with data analytics, enables organizations to create highly precise customer base segmentations and tailor products, services, and responses accordingly. As per David Krajicek of GfK Research, segmentation is the initial strategy that eventually snowballs into hyper-personalization.
Fast Moving Consumer Goods (FMCGs) and service companies that have leveraged segmentation for several years are beginning to deploy advanced and sophisticated big data techniques like real-time micro-segmentation of customers to yield better results from promotions and advertising.
Example 1:
Let’s say you work for a US company selling air conditioners and wish to prompt your audience to purchase more from your eCommerce store. Let's also assume that the weatherman has warned about a heatwave in certain regions. Thanks to micro-segmentation, you can profile customers who will be most affected and personalize their experience by offering coupons and free shipping for cooling devices!
Example 2:
Netflix, one of the most popular Over The Top (OTT) platforms, was groundbreaking when it deployed AI-powered algorithms to predict what a specific user might enjoy watching next. This prediction was based on the user’s watch history, search history, demographics, ratings, and preferences. YouTube has taken this even further with its “suggested videos,” which account for an astounding 70% of the videos consumed on the platform. Amazon, Tiktok, et al, adopted this personalization approach with much success.
Done properly, you can outmaneuver competitors.
2.Improving Decision-Making Powered By Predictive Analytics
Navigating a data-driven business world where swift and accurate decisions are made based on data-driven insights rather than a gut feeling is not easy. Predictive analysis can provide a glimpse into the future by predicting outcomes. Such predictions are possible today, thanks to modern analytics that can scan and analyze historical and real-time data to forecast trends. This significantly enhances decision-making, reduces risks, and uncovers important insights that would otherwise go unnoticed.
Example 1:
Harley-Davidson leverages predictive analytics through an AI program (called Albert) to reach out to potential customers.
Albert started analyzing the existing customer data from the customer relationship management (CRM) system to classify traits and behaviors of high-value ex-customers - those who have completed a purchase, viewed website content or were among the top 25 percentile of visitors in terms of time spent on the website.
Armed with key performance targets and creative content (headlines & visuals) provided by Harley-Davidson, Albert recognized lookalike customers who resembled the past customers and created micro-segments (small sample groups).
Albert ran test campaigns on these sample groups to ascertain each group's best visual and headline combinations. Once customers want to contact the sales personnel, they are guided through the sales process by the sales executives. Thanks to Albert - while customers get personalized service right when they consider making a purchase, the sales team can focus on exclusive serious customers.
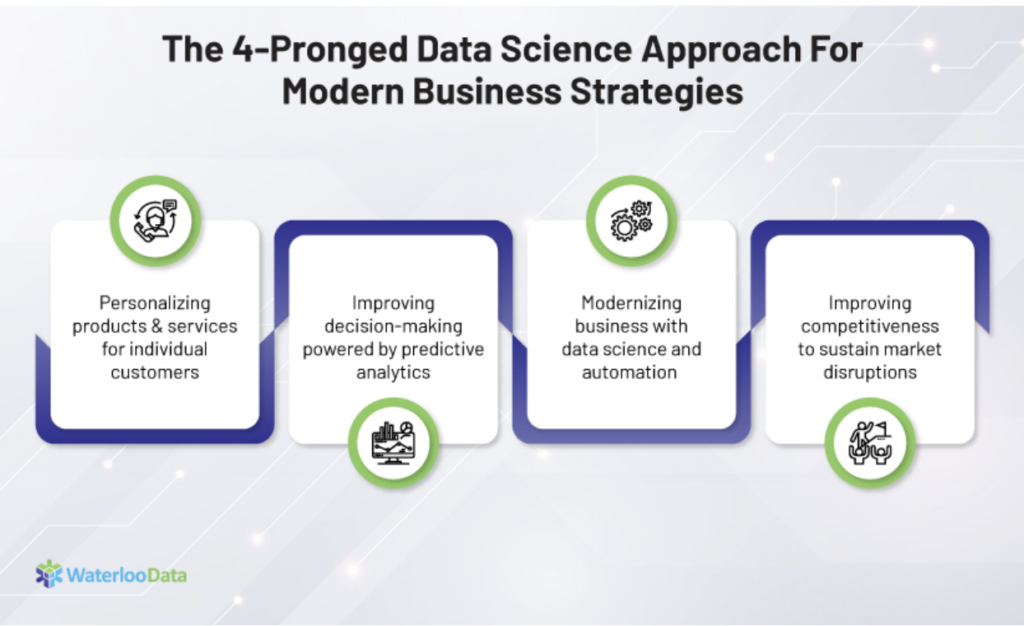
3.Modernizing Business with Automation
Automation has become an intrinsic part of most business strategies. Data science helps in the extraction, generation, and interpretation of data and is a precursor to automation that supports - high scalability, intelligent workflows, minimal downtimes, resource, time and cost savings.
For instance, when RPA (Robotic Process Automation) converged with data science, it was a game-changer. Suddenly, businesses could use machine learning to detect patterns in real-life processes and enhance them automatically by utilizing a technique known as process mining.
Process mining is a technique connecting data science and process management to discover, monitor and improve real processes like approving loans, receiving orders, etc., by extracting readily available knowledge from the event logs of information systems. The event log is an activity or interaction between people/software and a business IT system. This was probably the ultimate missing link towards “a fully automated enterprise” that numerous RPA tools were touting.
So there are two aspects to this:
- Identifying automation use cases: Process mining provides insights about processes that require improvement, harmonization, or automation.
- Automating the identified process: RPA is one of the tools for automating processes.
In simpler words, the former identifies automation opportunities, while the latter is an automation enabler.
Consider this example from the banking sector.
It takes a long time to process a mortgage loan in the United States. The approval process goes through credit checks, employment verification, repayment history, inspection, etc. Even the smallest of errors have the potential to slow down the process. Automation can address these concerns.
With the help of process mining, it becomes easier for a banking manager to formulate better strategies to fast-track mortgage loan approval while also looking out for customers who can be approached for other banking services. And RPA can implement the strategy to accelerate the loan approval process, minimizing human errors and ultimately reducing turnaround from weeks to minutes!
4.Improving Competitiveness To Sustain Market Disruptions
By leveraging customer data, businesses can gain a competitive edge and even thrive in times of market upheaval.
Pandora was the initial major player in digital music streaming; however, they are an example of a business that did it wrong. Even with the first-mover advantage, they failed to successfully compete with Spotify and Apple Music.
If we dive deeper, Pandora was a platform for users to discover new music that was musically similar to their taste. The service did not let users choose the exact songs they wished to listen to.
The Music Genome Project that powers the platform categorizes every track on hundreds of attributes (beat, pace, vocal styles, instruments, etc.). This assessment is then used to add similar tracks to the various stations. As per Pandora, it is the most exhaustive analysis of music ever undertaken.
While no one can deny the claim outright, Spotify may wink at that!
Spotify allows its listeners to play the songs they want to listen to. The service makes it easy to stream and share music (that users recognize and love). Spotify gradually implemented data mining(analyzing relationships between parameters like customer age, gender, tastes, etc., to generate meaningful insights that help design or improve business strategies accordingly) to understand its listeners’ even more thoroughly. Bottom line, Spotify used better data science and remains a leader.
Wrapping Up
As businesses face significant challenges to improve ROI in a disruptions-driven market, leveraging data science is necessary. Extracting maximum value from and creating disruptive strategies with the help of data science is the way forward.